How to Think About Your Oil Analysis Results
What do your results represent? We break down the basics of viewing your reports with a statistical mindset.
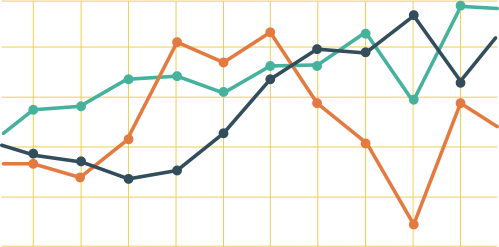
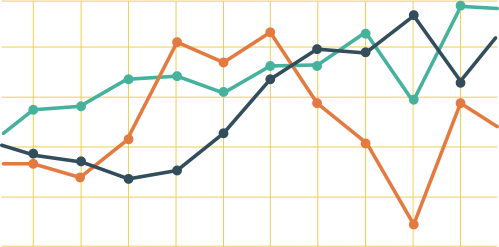
The end-result of any laboratory analysis is data.
Sometimes our customers will come to us with questions about their data. It can feel like they are being inundated with esoteric test names, values, and trends, and it isn’t clear what is important to focus on, and what is not.
Ultimately, it is up to the data analyst (one of our Certified Lubrication Specialists) to derive meaning from these results and communicate this information to the customer. However, gaining further understanding of the testing process, what these tests output, and what these data represent, will always serve to enrich, and empower, our clients’ oil condition monitoring programs.
What Is Data?
Although this question may seem philosophical, the differences between the concepts of ‘data’ and ‘information’ are important to your understanding of oil analysis results.
Strictly speaking, data is the raw, unorganized representation of measurements captured of an item of interest, usually compared to some standard.
The width of the desk that I am sitting at is an example of data, and its value varies depending on the standard of measure (e.g., the desk is 5 feet wide, 60 inches wide, and 1524 millimeters wide, depending on your choice).
If you run your hydraulic oil on laser particle count (ISO 4406) a single time, that represents a data point.
A data point, on its own, rarely provides information. Information is typically contrasted from data in that it provides understanding to its recipient. This is one reason that we always stress to our clients to pay close attention to the trend of the data, rather than isolated data points.
If you know you are filtering your hydraulic oil, but your ISO particle count is not diminishing, this gives you information that lets you know there is something potentially wrong with the filtration process. This realization is typically referred to, variably, as insight or knowledge.
It is very possible for a single, off-trend result to creep into your data set. If you immediately act on this data point, you could potentially engage in unnecessary, and costly, maintenance on your equipment. To avoid situations like these, we typically recommend our customers to resample any abnormal, off-trend result to confirm conditions.
Good analysis lies in the ability to separate the wheat from the chaff, providing the final link in the data -> information -> insight chain.
Accuracy, Precision, and Bias
When performing laboratory analysis, the goal is to be as accurate and precise as possible.
What is the difference, you ask?
As you can see from the picture above, if the center of the bullseye is the target value, the accuracy (or trueness) of a measurement would be how close that measurement is to the center.
Meanwhile, precision describes the level of variance seen in the results. High variance means that there is, on average, a large magnitude of difference between each individual result, relative to each other.
In the picture above, each bell curve is centered around the ‘true’ value. However, the level of precision represented by each curve differs wildly, with the red curve showing a relatively high level of precision, and the blue curve showing lower precision.
The average of the sum of the squared differences of each individual result from the mean (quite a mouthful) is the variance.
The standard deviation of a sample set is simply the square root of the variance. One thing that is nice about standard deviation is that it is expressed in the same units as the measurements, themselves.
Since we are still using the same units, we can say things like, “This measurement is 0.8 standard deviations from the mean”.
Why does this matter? Well, if we assume that our data set has a Gaussian (or normal) distribution, we can use the 68-95-99.7 rule to gain a quick, intuitive grasp on the likelihood that an individual measurement is valid.
Simply put, the 68-95-99.7 rule states that:
· 68% of a data set’s values will fall within 1 standard deviation from the mean.
· 95% of a data set’s values will fall within 2 standard deviations from the mean.
· 99.7% of a data set’s values will fall within 3 standard deviations from the mean.
What this indicates is that, if you get a result that falls outside of 2 standard deviations from the mean, then this result only has, at most, a 5% likelihood of being valid. If your result falls outside of 3 standard deviations from the mean, then it has, at most, a 0.3% chance of being valid (actually, you can usually say it has a 0.15% chance of being true, since that 0.3% is split on either side of the mean. This logic also applies to statement made regarding data points outside 2 standard deviations).
The data analysts at MRT are always on the lookout for these statistical irregularities, and we recheck any suspect data to confirm its accuracy.
So, what happens when your recordings are precise, but they are not accurate? This phenomenon is typically seen as an example of bias.
Sources of bias are many, but it is commonly caused by:
· Unrepresentative data sampling
· Systematic error in instrument measurements
· Faulty assumptions
The first example is also known as sampling bias. It is very easy for this form of bias to creep into your data.
Here is one practical way it could happen: if you always sample your oil from a port located behind a filter, where the filter lies in between the sample port and the rotating equipment, this can mask the detectable effects of machinery wear and therefore bias your results.
Likewise, sampling fluid that has been sitting near the bottom of the sump, near the drain, can significantly (and negatively) impact the cleanliness of the sampled fluid. The sampled oil is not actively lubricating the equipment, so it is not a representation of the true health of the system.
Sampling bias is the likely cause of a significant number of the abnormal sample reports we distribute.
Repeatability and Reproducibility
ASTM, ISO, and other standardization organizations typically list two measures of precision with their test methods: repeatability and reproducibility.
After getting an initial result, these two measurements provide a maximum range that subsequent results should conform to be considered valid.
Repeatability and reproducibility differ in how strict they are regarding the subsequent measurements.
Measurements taken by the same analyst, using the same equipment, under the same testing conditions, and on identical sample material, are subject to repeatability.
Measurements taken by a different analyst, using different equipment, under different testing conditions, or using non-identical sample material, are subject to reproducibility.
Essentially, repeatability represents within-run precision, while reproducibility represents between-run precision.
As you might expect, the repeatability threshold is often much narrower than the reproducibility threshold.
These values can significantly differ between tests, or even within the same test. The relative magnitude of the repeatability/reproducibility threshold reflects the overall level of precision possible when running that test.
For example, ASTM D6595 (Elemental Spectroscopy by RDE-OES) states the following reproducibility limits for zinc and phosphorus:
This table states that, if one laboratory runs a sample and receives 700 ppm for zinc and phosphorus, another laboratory could run that same fluid and receive a result that is 700 ± 176 ppm for zinc, and 700 ± 216 ppm for phosphorus. As far as ASTM D6595 is concerned, interlaboratory results for zinc of 700 and 870 ppm are identical.
The level of variance demonstrated here for phosphorus content is great enough that it could actually knock a passenger car engine oil out of API compliance when its formulation is perfectly conformant (we may have heard of this happening before from an anonymous lubricant manufacturer).
As demonstrated, knowledge of expected repeatability/reproducibility criteria are critical in laboratory operations. Recurring non-repeatable results may indicate instrument malfunction, improper training of personnel, or issues with testing standards.
Our Quality Process
As an ISO 17025 accredited laboratory, MRT has built a robust system for QA/QC.
All accredited methods are performed with NIST-traceable standards. Technicians are thoroughly trained to look out for many of the statistical irregularities we have discussed in this article.
All our equipment is strictly maintained with regular preventive maintenance and re-calibrations provided by qualified personnel.
Any non-repeatable results immediately trigger a quality assurance check, where the technician performs the analysis again with an appropriate standardized material.
The reference value of the standard should, optimally, be close to the expected value of the unknown material. Failing to adhere to this credo can lead to unfortunate consequences.
For example, if you suspect you have received an invalid result for an oil with a 320 cSt viscosity, it isn’t much good to confirm that your instrument is reading correctly at 22 cSt! It is entirely possible for the instrument to be inside calibration at one measurement range, and outside calibration at another.
Any non-repeatable results are immediately communicated to Lab Management, where a root cause analysis of the discrepancy is performed. Any instruments that are found to be outside of acceptable performance are taken out of commission until they can be repaired.
These systems help to ensure that our end-product, the sample report, can be relied on to provide accurate and actionable information to the client.
Conclusion
We hope that this summary of some of the numerical methodologies used in oil analysis has given you a fresh perspective on your results. Using these techniques, we can glean meaningful trends and forecast equipment condition into the future.
This allows our customers to employ predictive, rather than reactive, maintenance, which can make a huge difference on their bottom line by improving efficiency, preventing failures, and maximizing run-time.
Please feel free to reach out to us if you have questions.